Take a moment to imagine that you feel sick and you went to the hospital to see a doctor. The doctor attending to you is a junkie and high on drugs.
Will you trust his judgment or prescription?
Or you walked into a restaurant and ordered a cup of coffee. The waitress came back bringing a big-sized pizza with a bottle of Coke instead of the coffee you ordered because she mistook your order for someone else.
As far-fetched and unrealistic as this may sound, it's the same result you will get if your data is inaccurate or does not contain every element that guarantees data accuracy.
Data accuracy is not just a measure of correctness, but a reflection of the true real-world scenarios data is intended to represent.
Achieving such a level of precision involves meticulous processes across the data lifecycle, striking a balance between error-free information and the suitability of data collection, maintenance, and use.
This article categorizes data accuracy into various types, such as; validity, completeness, consistency, and more, illustrating the complex nature of what makes data accurate.
Definition of Data Accuracy
Data accuracy refers to the extent to which data correctly reflects the real-world situation it is supposed to represent. This means that data should be precise, correct, and free from errors or distortions, regardless of how the data was collected, recorded, or processed.
Data accuracy is important for making informed decisions, conducting reliable analyses, and maintaining the integrity of data-driven processes.
In practical terms, accurate data should represent the quantities or facts it is supposed to describe without deviations, omissions, or misinformation.
Achieving high data accuracy often requires strict data collection, validation, and maintenance practices, including error checking and quality control.
Types of Data Accuracy
When we talk about data accuracy, we have to cast our net far and wide because the concept of data accuracy encompasses various scopes, each focusing on a different aspect of the data's quality and suitability for use.
Let's take a look at some of the key types of data accuracy:
- Validity
Validity refers to the degree to which the data conforms to defined business rules, standards, or specific criteria. - Completeness
Completeness is about ensuring that all necessary data is present. Missing values can significantly impact the usefulness and reliability of data. - Consistency
This dimension checks whether the data does not contradict itself across the dataset or between datasets. Consistency is vital for maintaining the integrity of relationships within the data, such as; ensuring the same user is not listed with different birth-dates in different tables or databases. - Timeliness
Timeliness measures whether the data is up-to-date and available when needed. Data can lose its value if it is not provided in time for its intended use. - Uniqueness
Uniqueness ensures that each data record is distinct and that there are no duplicates within the dataset. Duplicates can lead to overcounting or misinterpretation of the data. - Reliability
Reliability pertains to the degree to which data is consistent and correct across multiple sources and over time. Reliable data can be trusted to make critical decisions. - Precision
Precision is about the level of detail provided by the data. Higher precision means more detailed and exact data.
Every type of data accuracy plays a strong role in ensuring that data meets the quality standards required for its intended use. Inaccurate data can lead to incorrect decisions, inefficiencies, and a lack of trust in the data systems. Therefore, it is a must to implement rigorous data management and quality control.
What Determines the Accuracy and Completeness of Organizational Data
When we talk about data accuracy, there are several key factors that influence the accuracy and completeness of data. These elements collectively are very fundamental, affecting its quality and, thus, the reliability of insights gotten from it. Some of the factors are:
Data Collection Methodologies
The techniques employed in gathering data play a crucial role in its eventual quality.
Precision in data capture—be it through automated sensors, direct user input, or third-party data providers—directly impacts its accuracy.
Errors during collection, such as; incorrect measurements or biased survey responses, can multiply through the data lifecycle, leading to biased analysis and decisions.
Data Entry and Integration Processes
Post-collection, the manner in which data is entered into databases or integrated from different sources significantly influences its accuracy and completeness.
Manual data entry, for instance, is prone to human error, whereas automated data pipelines can help mitigate such risks.
Additionally, integrating data from diverse sources requires meticulous mapping and transformation processes to ensure consistency and accuracy.
Data Governance Frameworks
A strong data governance strategy is important for maintaining data accuracy and completeness. This includes establishing clear policies for data quality standards, ownership, and stewardship.
By defining responsibilities and procedures for data management, organizations can create an environment that fosters accuracy and accountability in data handling.
Data Validation and Cleaning Techniques
Regularly employing data validation and cleaning techniques is essential for maintaining data quality. These practices help identify and fix errors, such as; duplicate records, missing values, or anomalies, ensuring the accuracy and completeness of the dataset.
Technology Infrastructure
The underlying technology infrastructure plays a foundational role in supporting data quality. Systems that facilitate real-time data processing, automated error detection, and seamless integration across platforms can dramatically enhance data accuracy and completeness.
As such, investments in modern data management technologies are vital for organizations aiming to improve their data quality.
Cultural Attitude Towards Data Quality
Lastly, an organization's culture greatly impacts data quality. Cultivating a data-centric culture, where data accuracy and completeness are valued and prioritized, encourages adherence to quality standards and processes.
Staff awareness and training on the importance of data quality further reinforce these values, leading to better data management practices across the organization.
Understanding and addressing the factors that determine data accuracy and completeness are critical for organizations.
By focusing on sound collection methods, rigorous data governance, advanced technology, and fostering a culture of data quality, organizations can chart a path toward high-quality, reliable data.
Understanding the Accuracy of Data
Data accuracy is a deciding factor that determines the reliability, credibility, and effectiveness of data analysis and decision-making processes.
High data accuracy is fundamental across various fields, including scientific research, healthcare, finance, engineering, and marketing, amongst others.
Several factors can influence the accuracy of data, including:
- Data Collection Method
The technique used for gathering data can significantly influence its accuracy. Direct, well-designed methods tend to yield more accurate data compared to indirect or flawed approaches. - Instrumentation
The tools and equipment used for measurement directly impact data accuracy. High-precision instruments usually provide more accurate measurements. - Human Error
Errors in data entry, interpretation, or processing can reduce data accuracy. Training, oversight, and automation can mitigate these errors. - Outdated or Obsolete Data
Information can become outdated or obsolete, making it less accurate for current use. Regular updates and validation are necessary to maintain data accuracy.
Strategies for Enhancing Data Accuracy
Improving the accuracy of data involves implementing several practices throughout its lifecycle:
- Quality Data Collection Practices
Using and designing clear and precise data collection processes, and adequately training personnel involved in data collection. - Data Verification and Validation
Implementing routines to check data for inconsistencies, outliers, or errors and validating data against known standards or benchmarks. - Data Cleansing
This involves identifying and correcting errors or inconsistencies in the data to ensure its quality. - Use of Advanced Technologies
Employing software and algorithms designed to minimize human error and bias in data collection, processing, and analysis. - Regular Data Audits
Conducting periodic reviews and audits of data to identify and correct any inaccuracies.
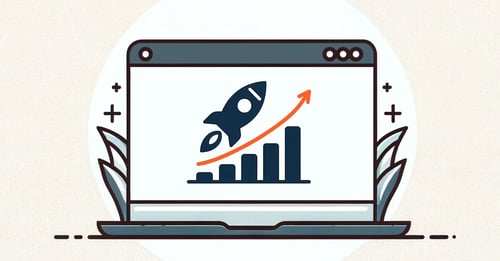
Interaction Between Accuracy and Completeness
Accuracy and completeness of data are two elements that can't be overlooked when it comes to achieving quality of information, influencing decision-making processes, research outcomes, and operational efficiency across various fields.
Both of these elements ensure that data effectively reflects the real-world conditions it's meant to represent. While they are related and often interchangeably used, they solve different aspects when it comes to data quality.
While accuracy focuses on how close the data is to its true values or the extent to which the data represents, completeness, on the other hand, concerns whether all required data is present and available for use. It assesses the extent to which the expected breadth and depth of information are fully represented in the dataset.
Achieving a balance between accuracy and completeness is vital. The aim is to gather as complete a dataset as possible without sacrificing accuracy.
Here are a few strategies:
- Validation Checks
Implement data validation techniques to ensure accuracy and detect anomalies or errors early in data entry processes. - ComprehensiveData Collection Strategies
Employ methods and technologies that enhance data collection processes, ensuring completeness without compromising data quality. - Regular Audits
Conduct periodic data audits to assess both the accuracy and completeness of datasets and identify areas for improvement.
What are the Importance of Completeness?
- Comprehensive Analysis
Completeness allows for a holistic view and analysis, enabling better insights and understanding of patterns, trends, and correlations. - Confidence in Results
With complete data, there’s higher confidence in the analysis results, predictions, and subsequent actions based on that data. - Regulatory Compliance
For many industries, maintaining complete records is not just beneficial but legally required to comply with regulatory standards and audits.
Completeness is a complementary aspect of data accuracy, indicating that all necessary data items are present.
Accuracy without completeness can lead to misleading insights, as the data might be correct but not representative of the whole picture.
Why is Consistency of Data needed in Data Accuracy?
Data consistency refers to the assurance that a dataset remains uniform across different stores or during various operations over time. This means that irrespective of where or how the data is accessed, it should maintain uniformity in format, structure, and value.
Consistency is especially crucial in places where data is replicated across multiple databases or where it undergoes frequent updates and modifications.
For example, in a distributed database system where data might be replicated across different servers for availability and redundancy, it is essential that an update made in one server is correctly reflected across all replicas.
Without consistency, different users may get different values for the same query, leading to confusion, erroneous data analysis, and faulty decision-making.
Some reasons why Consistency is Needed for Data Accuracy
- Ensures Reliability
Consistency helps in ensuring that the data across all platforms and systems is reliable. For instance, if financial records in a banking system show varying balances across different nodes, it could lead to severe discrepancies, affecting customer trust and compliance. - Improves Decision Making
Accurate data is crucial for making informed decisions. Consistency ensures that the data used in analysis and decision-making is uniform, which in turn guarantees that decisions are based on accurate and up-to-date information. - Facilitates Data Integration
With organizations increasingly using a diverse range of software solutions and databases, consistent data facilitates smoother integration and interoperability between different systems. This ensures that the insights derived from integrated data sources are accurate and reliable. - Enhances Data Quality
Consistency is a significant factor in the overall quality of data. High-quality data must be not only accurate and complete but also consistent. This is vital in contexts like customer data management, where inconsistencies can lead to poor customer experiences. - Supports Compliance and Auditing
Many industries are subject to strict regulatory requirements regarding data management. Consistent data ensures that organizations can meet these compliance requirements more easily and effectively.
Additionally, during audits, consistent data can simplify the review process, ensuring that records match across different reports and logs. - Boosts User Confidence
Inconsistent data can erode user confidence in an organization's data handling capabilities. When stakeholders can trust the consistency of the data, they are more likely to trust analyses, reports, and decisions derived from that data.
Data consistency plays a major role in ensuring data accuracy. It is not just about maintaining the same values across databases or systems; it's about ensuring the reliability, usability, and trustworthiness of the data.
What are the Roles Precision plays in Data Accuracy?
Precision refers to the granularity or detail level of the data. High precision is necessary for accuracy in systems requiring detailed analysis.
Enhancing precision involves using advanced data collection instruments, detailed data entry procedures, and analytics capable of handling fine-grained data.
Below are key roles that precision plays in enhancing data accuracy:
- Enhancement of Repeatability
Precision is about the consistency of data points . High precision implies that repeated data collection efforts under unchanged conditions produce closely similar results.
This repeatability is essential for validating experiments, surveys, or any form of data collection processes. Without precision, data may be unreliable, as variations in data collection can introduce uncertainties that detract from accuracy. - Improvement of Data Quality
High precision indicates that the data collection method is well-calibrated and performs consistently over-time. This consistency is vital for maintaining the quality of the data being collected, as it ensures that the information remains relevant and reliable for analysis.
Consequently, high-quality, precise data are more likely to be accurate, facilitating more informed decision-making. - Enablement of Fine Distinctions
In many fields, including science, engineering, and finance, making fine distinctions between datasets is critical. Precision allows for the detection of subtle differences or changes that may have significant implications. - Basis for Statistical Analysis and Modeling
Precision forms the foundation for rigorous statistical analysis and the development of predictive models. Highly precise data allow for more accurate statistical inferences, as the variability in the data is reduced.
This reduction in variability makes it easier to identify trends, patterns, and correlations, leading to more reliable and accurate analytical outcomes.
Accuracy and Reliability of Data
Data reliability refers to the consistency of data over time and across different measurements. Reliable data produces the same results under consistent conditions, indicating that the data collection methods, sources, and processes are stable and well-defined.
Reliability ensures that the data is dependable, and any decision or inference made based on such data can be replicated and validated by others.
Why Data Reliability is Important for Data Accuracy?
- Foundation for Accuracy
Reliable data forms the basis upon which accuracy is assessed. Without consistency in data collection and measurement, it becomes challenging to evaluate whether data is accurate or not.
Inconsistent data, no matter how close to the true value at a single point in time, will fail to provide a correct representation if it cannot be consistently reproduced. - Enhances Validity
Data reliability contributes to the validity of the data. Reliable data, by virtue of being consistent, improves the validity of conclusions drawn from it because it supports the idea that the data truly measures what it is supposed to. - Supports Decision-Making
Reliable and accurate data are vital for informed decision-making. In fields like medicine, finance, and policy-making, decisions based on unreliable or inaccurate data can have significant, sometimes disastrous, consequences.
Reliable data ensures that decisions made are based on consistent and accurate information, leading to better outcomes. - Facilitates Error Identification
When data is reliable, any deviations from expected patterns are more likely to indicate genuine trends or issues rather than inconsistencies in data collection or processing. This makes it easier to identify and correct errors, thereby enhancing the overall accuracy of the dataset. - Supports Replicability
In research and scientific studies, the ability to replicate findings is fundamental to advancing knowledge.
Reliable data is a prerequisite for replicability; without it, researchers cannot confidently validate studies or build upon existing knowledge, stunting scientific progress.
Without reliability, data accuracy is compromised, as the consistency required to validate and verify data as true or correct is missing.
Together, data reliability and accuracy ensure that data serves its purpose, whether it’s guiding strategic business decisions, or informing policy.
11 ways to Measure Accuracy of Data
Determining the accuracy of data is crucial across various professions, from scientific research to business analytics. Accurate data leads to valid conclusions, effective strategies, and reliable forecasts. Here are several key strategies to ensure or determine the accuracy of data:
- Source Verification
Evaluate the credibility of the data source. Use data from reputable sources known for accuracy, reliability, and relevance to your current needs or analysis. - Cross-Verification
Compare data from multiple sources to check for consistency. Discrepancies between sources can indicate inaccuracies. Use triangulation by employing different methods or data types to verify the same information. - Error Checking
Conduct error checking procedures, such as data validation rules, to ensure data meets specified criteria. Software tools or data cleansing methods can also be used to identify and correct anomalies, outliers, or incorrect data entries. - Statistical Sampling
Applying statistical sampling styles to assess data accuracy when reviewing the entire dataset is impractical. Ensure the sample is representative of the entire dataset for accurate extrapolation. - Data Audit
Perform periodic data audits to evaluate the accuracy and quality of data. This could involve reviewing the data collection methods, entry processes, and data storage mechanisms. - Understand the Data Collection Process
Have an in-depth understanding of how the data was collected. Factors like the data collection method, timing, and the individuals involved can affect accuracy. Look for potential biases in data collection and take steps to mitigate their impact. - Use Metadata
Review the metadata (data about the data) which includes information about data origin, date of creation, author, and changes over time. This can provide insights into the data’s reliability and potential limitations. - Engage Experts
When necessary, consult with subject matter experts to validate the accuracy of the data. Their expertise can provide insights that are not immediately obvious through data analysis alone. - Implement Quality Control Measures
Develop and adhere to strict quality control procedures throughout the data lifecycle - from collection to analysis. This reduces the likelihood of errors and enhances data accuracy. - Feedback Loops
Establish mechanisms for feedback on data quality and accuracy. Continuous improvement based on feedback can help in identifying inaccuracies and refining data collection and analysis processes. - Use Technological Tools
Leverage advanced data analytics and data validation tools. Machine learning algorithms, for instance, can be trained to identify data that deviates from expected patterns, highlighting potential inaccuracies.
Employing these strategies, organizations can greatly improve the accuracy of their data. Accurate data is the foundation of trustworthy analysis, informed decision-making, and robust predictions, making the effort to ensure data accuracy well worthwhile.
In conclusion, the quest for data accuracy is both a critical challenge and a remarkable opportunity for organizations aiming to leverage the full potential of their data.
The comprehensive roadmap outlined in this article stresses the importance of achieving data accuracy, highlighting the multifaceted nature of data types, the determinants of data quality, and the intertwined relationship between accuracy, completeness, and consistency.
FAQs About Data Accuracy
What ensures the accuracy of data?
There are several factors that determine data accuracy like Data Validation, Data Verification, sourcing data from Reliable Data Sources.
Why is data accuracy important?
Accuracy of data helps in informed decision making, efficiency and productivity. It also helps in building trust and credibility.
What is data quality and accuracy?
Data quality refers to the overall utility of a dataset as a function of its ability to be easily processed and used by end-users while data accuracy refers to the degree to which data correctly reflects the real-world situation it is supposed to represent.
Comments